Big data is one of the misunderstood (and misused) terms in today’s market. But with a clearer understanding of how to apply big data to business intelligence (BI), you can help customers navigate the ins and outs of big data, including how to get the most from their big data analytics.
What Do You Mean By “Big Data”?
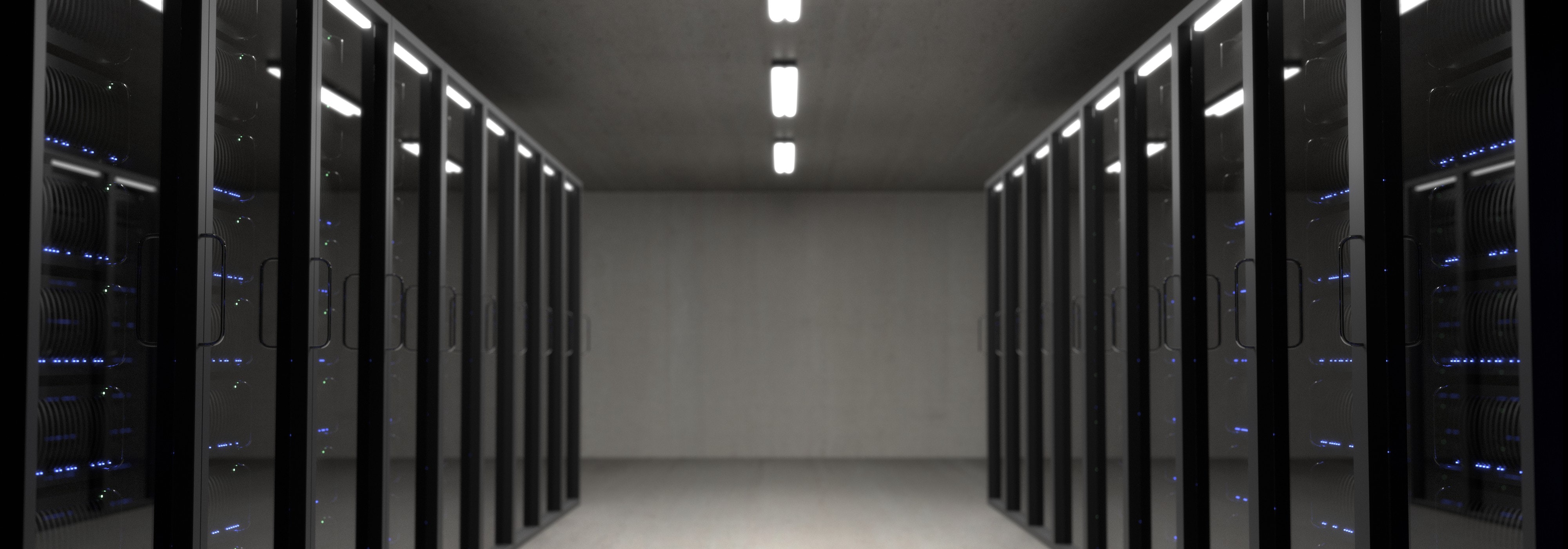
Big data can be applied to real-time fraud detection, complex competitive analysis, call center optimization, consumer sentiment analysis, intelligent traffic management, and to manage smart power grids, to name only a few applications. Big data is characterized by three primary factors: volume (too much data to handle easily); velocity (the speed of data flowing in and out makes it difficult to analyze); and variety (the range and type of data sources are too great to assimilate). With the right analytics, big data can deliver richer insight since it draws from multiple sources and transactions to uncover hidden patterns and relationships.
There are four types of big data BI that really aid business:
- Prescriptive – This type of analysis reveals what actions should be taken. This is the most valuable kind of analysis and usually results in rules and recommendations for next steps.
- Predictive – An analysis of likely scenarios of what might happen. The deliverables are usually a predictive forecast.
- Diagnostic – A look at past performance to determine what happened and why. The result of the analysis is often an analytic dashboard.
- Descriptive – What is happening now based on incoming data. To mine the analytics, you typically use a real-time dashboard and/or email reports.
Big Data Analytics in Action
Prescriptive analytics is really valuable, but largely not used. Where big data analytics in general sheds light on a subject, prescriptive analytics gives you a laser-like focus to answer specific questions. For example, in the health care industry, you can better manage the patient population by using prescriptive analytics to measure the number of patients who are clinically obese, then add filters for factors like diabetes and LDL cholesterol levels to determine where to focus treatment. The same prescriptive model can be applied to almost any industry target group or problem.
Predictive analytics use big data to identify past patterns to predict the future. For example, some companies are using predictive analytics for sales lead scoring. Some companies have gone one step further use predictive analytics for the entire sales process, analyzing lead source, number of communications, types of communications, social media, documents, CRM data, etc. Properly tuned predictive analytics can be used to support sales, marketing, or for other types of complex forecasts.
Diagnostic analytics are used for discovery or to determine why something happened. For example, for a social media marketing campaign, you can use descriptive analytics to assess the number of posts, mentions, followers, fans, page views, reviews, pins, etc. There can be thousands of online mentions that can be distilled into a single view to see what worked in your past campaigns and what didn’t.
Descriptive analytics or data mining are at the bottom of the big data value chain, but they can be valuable for uncovering patterns that offer insight. A simple example of descriptive analytics would be assessing credit risk; using past financial performance to predict a customer’s likely financial performance. Descriptive analytics can be useful in the sales cycle, for example, to categorize customers by their likely product preferences and sales cycle.
As you can see, harnessing big data analytics can deliver big value to business, adding context to data that tells a more complete story. By reducing complex data sets to actionable intelligence you can make more accurate business decisions. If you understand how to demystify big data for your customers, then your value has just gone up tenfold.
It works very well for me
It works quite well for me